This study presents two novel methods for fusing RGB and Depth images with LIBS using Deep Learning models. The first method is a single-output model that combines LIBS UNET and two DenseNets in a late fusion framework. The second method is a multiple-output model that uses the structure of the single-output model to enhance learning and avoid overfitting. The first sorting task is separating Cast and Wrought (C&W) aluminum. The second is the division of the post-consumer aluminum scrap into three commercially interesting fractions. The single-output model performs best for separating C&W, with a Precision, Recall, and F1-score of 99%. The multiple-output model performs best for classifying the three selected commercial fractions, with a Precision, Recall, and F-score of 86%, 83%, and 84%. The presented data fusion method for LIBS and computer vision images encompasses the great potential for sorting post-consumer aluminum scrap.
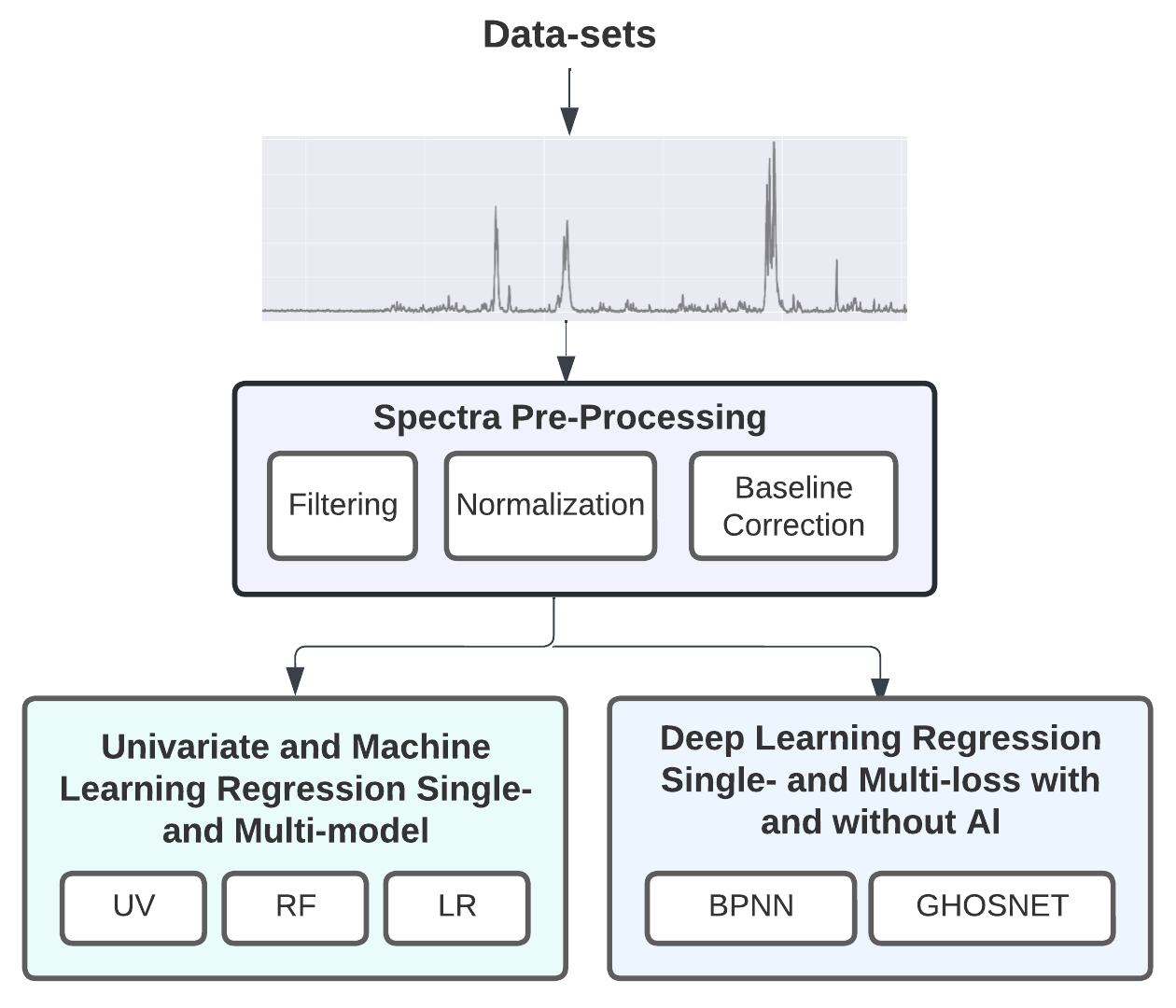